Platform architecture derisked
Delivering a future-proof framework for AI-assisted auditing
01. Question
All too often, innovative business strategies tend to raise more questions about their long-term impact than the immediate issues they seek to address. One of our clients reached out to us to solve this classic conundrum for them.
In 2020, the Flemish government enlisted our services to assist in a tendering process for one of their flagship projects. As detailed in our previous case study on the topic, this ambitious undertaking aimed to rewrite the rules of auditing in government agencies by integrating AI-assisted processes for auditors. The initial RFP that we’d assisted on, was set to mark the start of a new, AI-based auditing process for our client. Since then, the artificial intelligence landscape has shifted and underlying algorithms have made significant strides. A crucial question for our client remained, however: how best to take advantage of the opportunities presented by data mining, AI and machine learning, when uncertainty still loomed over future technological advances? Our client was looking for a solid development and implementation approach.
That’s why we were called upon to craft a forward-looking blueprint for our client’s digital architecture, in close collaboration with their implementation partner. We’d need a robust, future-proof design that could not only keep pace with the latest developments in AI algorithms and capabilities, but also stand the test of time and accommodate future AI advancements. Our objective? To formulate a well-defined end-state architecture over a six-year horizon, in turn mitigating potential risks and enabling the implementation partner to focus on their work.
02. Process
Creating a sustainable environment requires in-depth technical expertise, an intimate understanding of the client’s auditing environment, and key insights from their key personnel. Drawing on this knowledge, we embarked on a series of short iterations to formulate the foundational components comprising our first-stage architecture. For each of these building blocks, we crafted a comprehensive proposal for its inner workings, which formed the basis for detailed discussions with the client’s implementation partner. Our primary objective was to minimise risks associated with each individual building block, ensuring a seamless implementation process for the development partner.
To streamline our collaborative efforts, we scheduled semiweekly meetings with the client’s key workers. This approach allowed us to tap into their expertise, which we then leveraged in the architecture.
“It pays off to think ahead. A limited twelve-week effort ensures a solid plan of action for the next six years.”
03. Insights
Our work yielded four key insights that can be applied to similar projects of this nature, irrespective of their industry:
1: Avoid chasing the Everything Model
Auditing inherently entails scrutinising for inadvertent errors and deliberate missteps in filed applications. While the prospect of constructing an all-encompassing data model to churn out a verdict on a claim might sound tempting at first sight, this approach often proves impractical. The initial challenges lie in sourcing sufficient training data and designing the appropriate features – hurdles that can stall even the most ambitious of modelling tasks. Instead, our approach involved leveraging the existing knowledge and expertise of the client’s employees to compartmentalise each auditing challenge. We used this insight to construct a series of small-scale tests and checklist-based validations to assist auditors, rather than attempting to devise an all-embracing model. The output of this series of tests captures the risk associated with each claim, and is subsequently used in an AOQL sampling process to ensure the materiality bounds of audit are respected.
2: Base solutions on requirements, not templates
Solving problems while adhering to a fixed template or methodology doesn’t invariably yield the best results in the long run. Guided by our client’s long-term objectives, we meticulously devised the necessary requirements. Subsequently, we matched these with the appropriate mathematical model tailored for the specific task at hand. We embraced classic statistical approaches, including Bayesian networks, where suitable, resorting to machine learning (e.g. random forest decision trees or logistic regression) when necessary. If the only tool you have is a hammer, it is tempting to treat everything as if it were a nail. Fortunately, artificial intelligence is just one tool in our belt.
3: Leverage the appropriate language models
Generative AI and language prediction models like OpenAI’s GPT-4 and Meta’s Llama 2 have democratised the natural language processing needs of organisations in unprecedented fashion. At the same time, our role mandates finding the ideal model for each task, without undue complexity. To mitigate potential challenges posed by language models and to increase accuracy, we harnessed the client’s own expertise to engineer simple end prompts that would reduce complexity as much as possible. We also evaluated different language models tailored to niche purposes (including Google’s BERT), ensuring a perfect fit for every subtask and building block.
4: Factor in historical performance while remaining open for future improvements
In projects of this nature, it’s imperative to consider historical data and expert knowledge in an attempt to uncover hidden drivers that play a pivotal role in feature engineering. Simultaneously, as technologies evolve and business circumstances take unpredictable turns, a number of so-called ‘unknown unknowns’ will emerge over time. This means that the optimal solutions for each building block in our client’s architecture will also evolve. It’s paramount to recognise this inevitability right from the start and prepare for future changes by safeguarding the architecture and system’s capacity for continuous learning and improvement. We achieved this in three ways. First, the predictive power of all models are monitored continuously via cross-entropy, highlighting when certain models must be redesigned. Secondly, we adopted a modular approach to architectural design. Individual components can be seamlessly replaced without compromising the application’s overall performance. Thirdly, we developed a detection mechanism that relies on anomaly detection, ranging from outlier inspection after clustering the data to language-based anomaly detection, and other tell-tale patterns to flag cases for further human review.
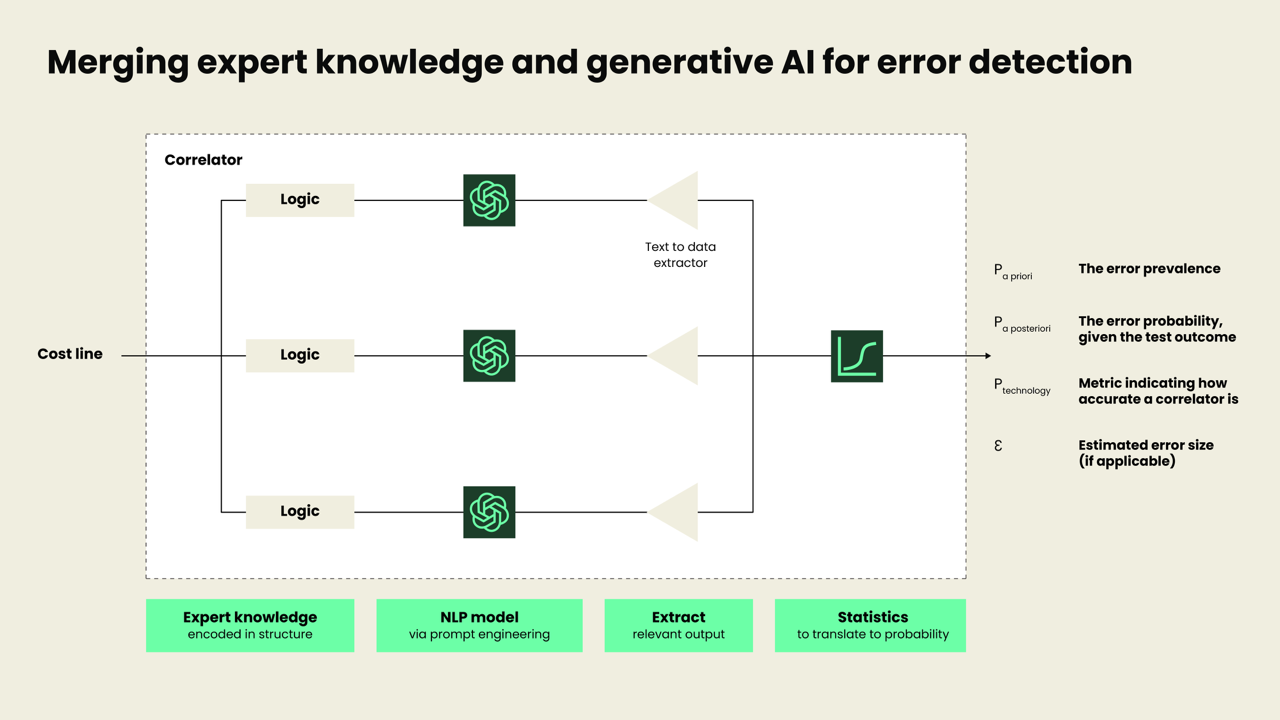
04. Results
The outcome? A comprehensive and future-proof architecture, complete with derisked building blocks, primed for immediate implementation. We’ve enriched the architecture with detailed mathematical explanations, caveats, and alternative language models. This way, our twelve-week effort ensures a solid plan of action for the next six years. As a follow-up to this project, we are currently also involved in rapid prototyping for parts of our work. This not only reduces risks associated with individual components, but also serves as a guide for our implementation partner.
Accelerating digitalisation isn’t limited to the public sector alone; it holds potential for a wide range of governmental and private organisations. A practical AI strategy framework drives progress, cuts costs and enables teams to focus on their core strengths. Through thorough data analysis and strategic thinking, we make sure to generate concepts that are fundamentally solid, achievable and well-prepared for the future.
Want to know more about this case?
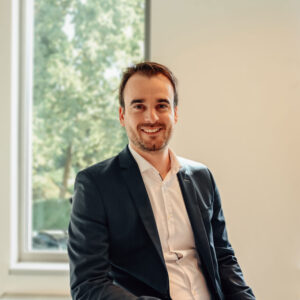